Exploring the Capabilities and Future Potential of Multi-Modal LLMs
- 5 minutes read - 869 words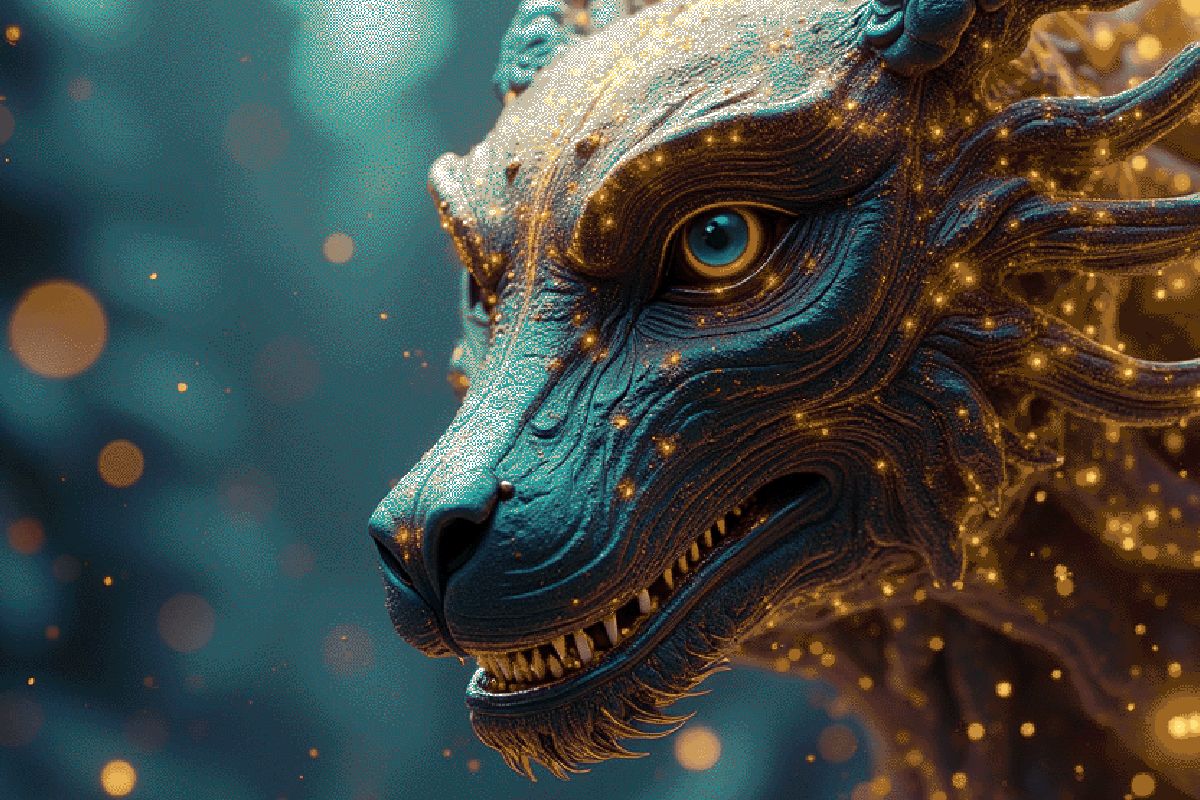
Table of Contents
In the rapidly evolving landscape of artificial intelligence, multi-modal large language models (LLMs) are emerging as a groundbreaking innovation. These advanced AI systems are designed to process and generate information across various formats, including text, images, audio, and video. By comprehending and producing content that combines different types of data, multi-modal LLMs offer unparalleled adaptability and power for a wide range of applications.
Key Features and Capabilities
Comprehensive Understanding
One of the standout features of multi-modal LLMs is their ability to simultaneously process and understand inputs from multiple formats. For instance, these models can analyze an image, produce a text description, interpret it, and even generate a corresponding image. This capability allows for a more detailed and contextually rich understanding of the data, making them invaluable in fields such as content creation, data analysis, and automated reporting.
Cross-Modal Generation
Multi-modal LLMs excel in creating outputs in one format based on inputs from another. For example, they can generate an image from a textual prompt or produce text [describing the content of a video]https://unimatrixz.com/topics/story-telling/scene-extraction-from-movie/. This feature is particularly beneficial in applications like content creation, virtual assistants, and automated reporting. Imagine a world where a virtual assistant can not only understand your spoken commands but also generate images or videos based on your descriptions. This level of cross-modal generation opens up new possibilities for creative expression and efficient communication.
Enhanced Interaction
Multi-modal LLMs significantly improve human-computer interaction by utilizing multiple types of input. Users can interact with these models using a mix of speech, text, and visual inputs. This capability enhances the user experience in chatbots, virtual reality (VR), and augmented reality (AR) applications. For instance, in a VR environment, users can describe a scene verbally, and the model can generate a corresponding visual representation, making the interaction more intuitive and immersive.
Comparison to Other Approaches
CLIP Scoring
CLIP scoring is a method for evaluating an image’s aesthetics and obtaining a description of its content. This technique leverages the Contrastive Language-Image Pre-training (CLIP) model, a neural network trained on a large dataset of image-text pairs. By comparing the similarity between an image and its potential descriptions, CLIP scoring can provide insights into an image’s visual appeal and contextual information.
While CLIP scoring is effective for evaluating and describing images, it is limited to a single modality—images and text. Multi-modal LLMs, on the other hand, can handle a broader range of data types, including audio and video, making them more versatile and powerful for complex tasks.
Dedicated Models (like LLaVA)
LLaVA (Large-scale Language-and-Vision Assistant) is an end-to-end trained multimodal model that automatically generates image descriptions by integrating a vision encoder with a large language model, Vicuna. This model was specifically developed to enable comprehensive visual and language understanding, allowing it to process and generate text based on visual inputs, focusing on image description generation.
LLaVA represents a significant advancement in the field of multimodal AI, but it is primarily focused on image description generation. Multi-modal LLMs, however, are designed to handle a wider array of tasks, including generating images from text, producing text from audio, and more. This broader capability makes multi-modal LLMs a more versatile tool for various applications.
Applications and Future Potential
The applications of multi-modal LLMs are vast and varied. In the field of content creation, these models can assist artists and designers by generating visual content from textual descriptions. In healthcare, they can analyze medical images and generate reports, aiding in diagnosis and treatment. In education, multi-modal LLMs can create interactive learning materials that combine text, images, and audio, making learning more engaging and effective.
Looking ahead, the potential of multi-modal LLMs is immense. As these models continue to evolve, they will likely become integral to our daily lives, enhancing communication, creativity, and efficiency across numerous sectors. The ability to understand and generate content across multiple modalities opens up new avenues for innovation and problem-solving, making multi-modal LLMs a key technology for the future.
Conclusion
Multi-modal large language models represent a significant leap forward in the field of artificial intelligence. Their ability to process and generate information across various formats makes them incredibly adaptable and powerful for a wide range of applications. From content creation to healthcare and education, these models offer unparalleled capabilities that enhance human-computer interaction and open up new possibilities for innovation. As we continue to explore the potential of multi-modal LLMs, it is clear that they will play a crucial role in shaping the future of AI and its applications.