AI Mood Guidance: A Deep Dive into Image Generation
- 4 minutes read - 732 words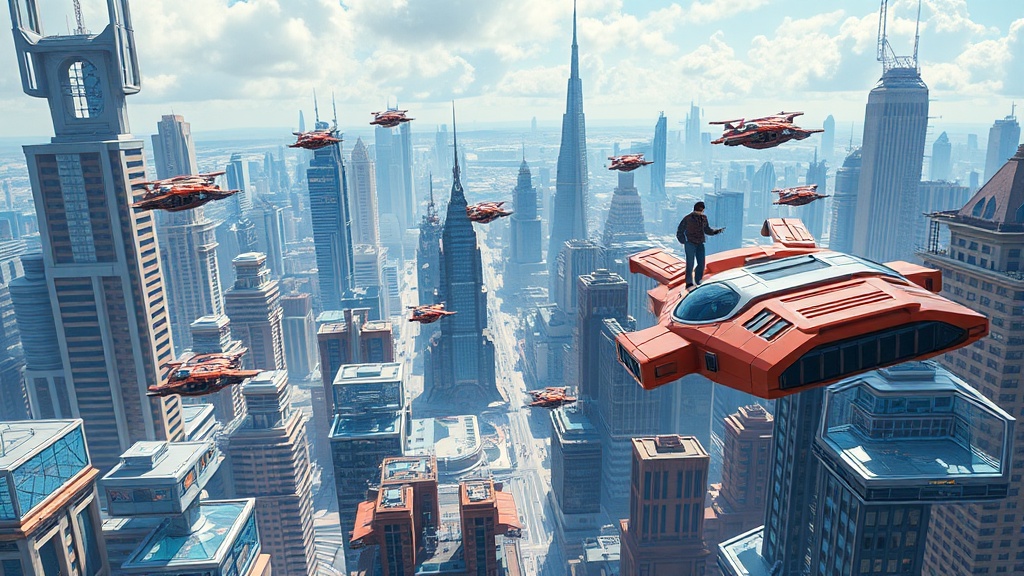
Table of Contents
The ability to generate images that evoke specific moods is a crucial aspect of AI image generation. This blog explores the performance of various AI models in capturing the intended mood in their outputs. We analyze data from a range of models, focusing on their strengths and weaknesses in translating mood into visual form. This analysis provides valuable insights into the current state of AI image generation and its potential for creative applications.
Top and Bottom Performers in Mood Guidance
- Imagen-v2 and Midjourney consistently demonstrate strong mood guidance, with scores ranging from 0.20 to 0.22. This suggests their ability to effectively translate the desired mood into the generated images.
- Imagen-v3-fast struggles with mood guidance, with scores consistently below 0.20. This indicates a weaker ability to capture the intended mood in its outputs.
- Flux-pro shows moderate performance, with scores hovering around 0.10. This suggests a potential for improvement in capturing the desired mood.
Image Examples
Cyberpunk City Divided: A Tale of Two Worlds
Mood Guidance : 0.00
dramatic-styles Split Screen: Focus, excitement ; A gamer’s hands furiously manipulating a controller; close-up; Gaming; a vibrant, futuristic cityscape projected on a screen; cinematic
A City Under a Gloomy Sky
Mood Guidance : 0.05
Stylized: Energetic and lively ; A panoramic view of a bustling city; long shot; Tourism; A vibrant and colorful cityscape; cinematic
Lost in the Code: A Hand Navigates the Digital Landscape
Mood Guidance : 0.09
camera-positions Dutch angle: Intense, focused, competitive ; A gamer’s hands, furiously tapping buttons on a controller; close-up; Gaming; A brightly lit room with flashing lights and screens; cinematic
The Finger on the Trigger
Mood Guidance : 0.09
close-up close-up: intense, focused ; A gamer’s hand, fingers flying across a keyboard, eyes locked on the screen; close-up; gaming; a dimly lit room with neon lights reflecting on the screen; cinematic
Lost in the Concrete Jungle: A Solitary Figure Navigates a Bleak Urban Landscape
Mood Guidance : 0.13
Desaturation: Solitary, powerful ; A lone figure walking through a deserted city street, the buildings towering above them; long shot; Heroism; a sense of isolation and determination; cinematic
Steel Giant in a Blue Abyss
Mood Guidance : 0.15
camera-positions Crane shot: exuberant, celebratory ; A hero celebrating a victory; crane shot; gaming; fantasy world; cinematic
Lost in the Neon Rain: A Cyberpunk Gamer’s Immersive World
Mood Guidance : 0.20
camera-positions Steadicam shot: Intense, focused ; A gamer’s hands manipulating a controller; close-up; Gaming; a vibrant, futuristic cityscape on the screen; cinematic
City Lights, Cozy Vibes: Friends Enjoy a Night Out
Mood Guidance : 0.21
Stylized: Social and celebratory ; A group of friends enjoying a meal at a restaurant with a view; medium shot; Tourism; A bustling city street with vibrant lights; cinematic
Soaring Through the Neon Skyline
Mood Guidance : 0.21
Rule of Thirds: Dynamic, exhilarating ; A gamer’s avatar soaring through a vibrant, futuristic cityscape; Wide shot; Gaming; A neon-lit, futuristic city with flying vehicles; cinematic
Neon Riders: A Futuristic Mystery Unfolds
Mood Guidance : 0.22
Cyberpunk: exciting, exhilarating ; A group of friends racing through a neon-lit cityscape on hoverboards; long shot; Adventure; futuristic cityscape with towering skyscrapers and flying vehicles; cinematic
Implications for Creative Applications
The findings highlight the importance of selecting the right AI model for specific creative tasks. Models like Imagen-v2 and Midjourney are well-suited for projects requiring a strong emphasis on mood and emotional impact. However, for tasks where mood is less critical, models like Imagen-v3-fast might be sufficient. Further research and development are needed to improve the mood guidance capabilities of all AI models, enabling them to generate even more expressive and emotionally resonant images.
Conclusion
This analysis reveals that AI models vary significantly in their ability to capture the intended mood in generated images. While some models excel in this area, others struggle to translate mood effectively. These findings have significant implications for creative applications, emphasizing the importance of selecting the right model for specific tasks. As AI image generation continues to evolve, we can expect further advancements in mood guidance, leading to even more expressive and emotionally engaging visual outputs.