Chatting with GPT-4 on Gödel, Popper, and AI alignment
- 38 minutes read - 8014 words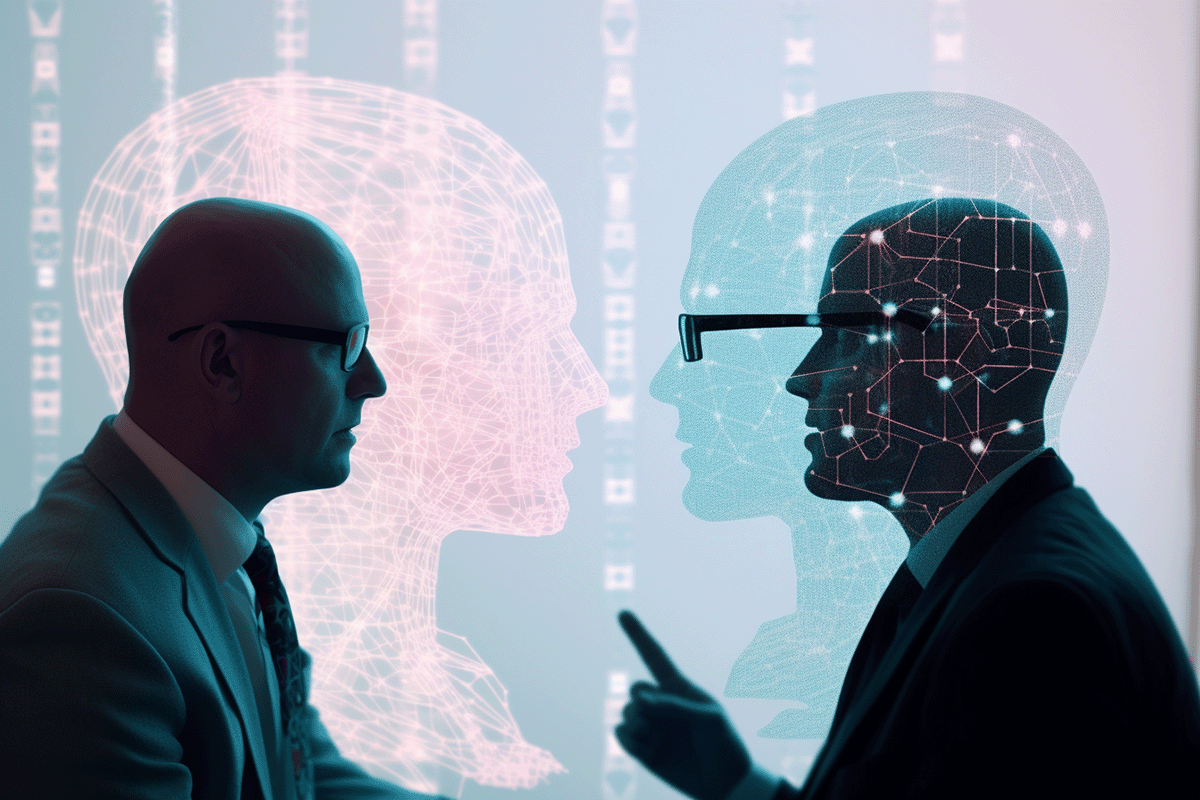
Table of Contents
Chat with GPT-4 about Kurt Gödel’s incompleteness theorems, Karl Popper, inductive reasoning, and AI alignment.
The text was left unredacted, also no grammar and spelling corrections; the text is presented raw.
Interview Text (very long)
[Interviewer] If humans and AI are two different axioms will Kurt Gödel‘s incompleteness theorems prevent an alignment between humans and AI
[ChatGPT, GPT-4, Late March 2023] Gödel’s incompleteness theorems are foundational results in mathematical logic that show there are inherent limitations to any formal system that is expressive enough to describe arithmetic. They state that, for such a system:
It is impossible to prove its own consistency. There will always be true statements within the system that cannot be proven. However, Gödel’s theorems don’t directly apply to the alignment of humans and AI because they are specifically about formal mathematical systems, not about the complex interactions between humans and AI systems.
The concept of alignment in AI refers to the development of artificial intelligence systems that understand, predict, and respect human values and preferences, so they can act in ways that are beneficial to humanity. Achieving alignment is a challenging task due to the complexities involved in understanding human values, preferences, and biases. It is also difficult to ensure that AI systems generalize well to new situations and do not exhibit unintended consequences or undesirable behavior.
The challenge of AI alignment is not primarily about the limitations of formal systems as described by Gödel’s theorems, but rather about understanding and modeling the intricacies of human values, preferences, and social dynamics, and incorporating them effectively into AI systems.
Nevertheless, Gödel’s theorems do remind us that there may be inherent limitations in our ability to formally prove certain properties of AI systems or the interaction between humans and AI. In practice, AI alignment is likely to be an iterative and empirical process, where we continually improve our AI systems based on feedback and experience, rather than aiming for a one-time, perfect, and formally provable solution.
[Interviewer] Aren’t human understanding and modeling the intricacies of human values, preferences, and social dynamics an axiom or can be seen as an axiom
[ChatGPT, GPT-4, Late March 2023] An axiom is a statement or proposition that is accepted as true without proof, serving as a starting point for a logical system. In the context of human values, preferences, and social dynamics, it might be more accurate to say that these are complex, multifaceted phenomena rather than axioms.
However, one could argue that certain basic principles or assumptions about human behavior, values, or preferences could be treated as axioms when designing AI systems or developing theories about human-AI interaction. For example, some researchers in AI alignment and ethics might assume that human well-being is an essential goal that AI systems should strive to optimize.
But even if we treat some aspects of human values or preferences as axioms, this does not directly invoke the implications of Gödel’s incompleteness theorems. As mentioned earlier, Gödel’s theorems apply to formal mathematical systems that are expressive enough to describe arithmetic. The challenge of AI alignment is more about understanding and modeling complex human behavior, values, and preferences, rather than proving the consistency or completeness of a formal mathematical system.
It is important to recognize that the study of human values, preferences, and social dynamics is a multifaceted endeavor, drawing from various fields such as psychology, sociology, anthropology, and cognitive science. These fields employ a wide range of methods and techniques to build models and theories about human behavior, which can inform the development of AI systems that are aligned with human values. While some basic principles or assumptions might be treated as axioms, the overall process is typically more complex and nuanced than a strict axiomatic system.
[Interviewer] Doesn’t that mean that AI alignment is arbitrary and random instead of a provable formalism
[ChatGPT, GPT-4, Late March 2023] AI alignment is not arbitrary or random, but it is also not a provable formalism in the same sense as mathematical theorems. AI alignment is a complex, interdisciplinary challenge that involves understanding human values, preferences, and social dynamics and incorporating them into AI systems to ensure that they behave in ways that are beneficial to humanity.
Although AI alignment may not be reducible to a set of provable axioms or theorems like those in formal mathematics, it is grounded in empirical research, ethical considerations, and practical engineering. The goal is to create AI systems that are reliable, safe, and beneficial, based on our best understanding of human values and preferences, and informed by insights from various fields such as psychology, sociology, anthropology, and cognitive science.
AI alignment is an iterative process that relies on continuous learning, feedback, and improvement. As AI systems become more capable and autonomous, it is crucial to ensure that they align with human values and intentions, which may involve refining and adapting our models of human preferences and behavior. This process may not have the same level of formalism as a mathematical proof, but it is a necessary and essential part of responsible AI development.
In summary, AI alignment is not arbitrary or random, but it is also not a provable formalism like those in mathematics. Instead, it is a complex, interdisciplinary challenge that requires an understanding of human values, preferences, and social dynamics, as well as the development of AI systems that are designed to be safe, reliable, and beneficial.
[Interviewer] AI alignment is an unprovable set of more or less understood rules and agreements
[ChatGPT, GPT-4, Late March 2023] AI alignment can be seen as a set of principles, guidelines, and objectives that researchers and engineers work toward to ensure AI systems are safe, reliable, and beneficial. These principles and guidelines are informed by our understanding of human values, preferences, and social dynamics, as well as technical considerations and ethical concerns.
While AI alignment might not be a provable formalism like a mathematical theorem, it is a vital aspect of responsible AI development. The process of aligning AI systems with human values is an ongoing, iterative process that requires continuous learning, feedback, and improvement. It is not a static set of rules or agreements, but rather a dynamic field that evolves as our understanding of human values, preferences, and AI capabilities grows.
The AI alignment challenge involves collaboration across multiple disciplines, including computer science, psychology, sociology, anthropology, and cognitive science, among others. By drawing from various fields and perspectives, researchers can develop a more nuanced understanding of human values and preferences and work towards designing AI systems that respect and align with them.
In conclusion, AI alignment is an essential aspect of AI research and development, which aims to ensure that AI systems are safe, reliable, and beneficial to humanity. It is not a provable formalism, but rather an interdisciplinary challenge that requires understanding and incorporating complex human values, preferences, and social dynamics into the design and development of AI systems.
[Interviewer] Is AI alignment grounded in provable truth (assuming logic), or a loose set of agreements which also depend on the socio-political context
[ChatGPT, GPT-4, Late March 2023] AI alignment is not grounded in provable truth like formal mathematical or logical theorems. Instead, it is a combination of principles, guidelines, and objectives informed by our understanding of human values, preferences, social dynamics, technical considerations, and ethical concerns. It is an interdisciplinary challenge that requires collaboration across multiple fields, including computer science, psychology, sociology, anthropology, and cognitive science, among others.
While AI alignment might not be reducible to a set of provable axioms or theorems, it is not solely a loose set of agreements either. The field of AI alignment has produced various frameworks and methodologies to guide the design and development of AI systems that are safe, reliable, and beneficial to humanity. Researchers in AI alignment strive to create AI systems that generalize well to new situations, avoid unintended consequences, and respect human values and preferences.
It is true that AI alignment is influenced by the socio-political context, as human values and preferences are diverse and shaped by cultural, historical, and societal factors. Different societies might prioritize different values or have different ethical concerns, which can affect how AI systems are designed and aligned. AI alignment should ideally take these diverse perspectives into account and aim to develop AI systems that are respectful and beneficial to a wide range of stakeholders.
In conclusion, AI alignment is neither grounded in provable truth like formal logical systems nor solely a loose set of agreements. Instead, it is an interdisciplinary field that combines principles, guidelines, and objectives informed by our understanding of human values, preferences, social dynamics, technical considerations, and ethical concerns. It is an ongoing, iterative process that is influenced by the socio-political context and aims to ensure that AI systems are safe, reliable, and beneficial to humanity.
[Interviewer] AI alignment isn’t about truth, assuming that there is only a single truth even considering a multi dimensional multiverse and multiple time dimensions
[ChatGPT, GPT-4, Late March 2023] AI alignment is not about discovering a single, universal truth. Instead, it is a process of designing and developing AI systems that understand, predict, and respect human values, preferences, and social dynamics in a way that is beneficial to humanity. The challenge of AI alignment lies in understanding the complexities of human values, preferences, biases, and behavior, and incorporating these insights into AI systems to ensure they act in ways that are aligned with human intentions.
Human values and preferences can be diverse, context-dependent, and influenced by various factors, such as cultural, historical, and societal backgrounds. AI alignment should take these diverse perspectives into account and aim to create AI systems that are beneficial and respectful to a wide range of stakeholders.
The concept of a “single truth” is less relevant in the context of AI alignment, as human values and preferences are not fixed, objective truths but rather complex, multifaceted phenomena that can vary across individuals, societies, and time. The goal of AI alignment is not to discover a singular truth about human values but to develop AI systems that can understand and respect the diverse values and preferences of the people they interact with and serve.
In conclusion, AI alignment is not about discovering a single truth or objective reality, but rather about understanding and modeling the intricacies of human values, preferences, and social dynamics and incorporating them effectively into AI systems so they can act in ways that are beneficial to humanity. This challenge requires an interdisciplinary approach that takes into account the complexities and diversity of human perspectives, rather than focusing on a singular truth.
[Interviewer] That means depending on the socio-political context AI alignment means different things? Like a libertarian AI alignment, conservative AI alignment, socialist AI alignment, etc
[ChatGPT, GPT-4, Late March 2023] AI alignment, at its core, aims to create AI systems that understand, predict, and respect human values, preferences, and social dynamics in a way that is beneficial to humanity. However, human values and preferences are diverse and can be influenced by various factors, including socio-political context. In different socio-political environments, people may prioritize different values, and AI alignment could consequently take on different aspects.
For example, a libertarian AI alignment might emphasize individual autonomy and freedom, while a conservative AI alignment might prioritize stability, tradition, and social order. Similarly, a socialist AI alignment might emphasize social justice, equality, and collective well-being.
In practice, AI alignment should ideally take into account the diverse values and preferences of individuals and communities across different socio-political contexts. This is a challenging task because it requires understanding and balancing a wide range of perspectives, and may involve making difficult trade-offs or navigating ethical dilemmas.
It’s important to note that AI alignment should not be reduced to specific socio-political ideologies. The ultimate goal is to create AI systems that are safe, reliable, and beneficial to humanity as a whole. Researchers and developers should strive to ensure that AI systems are designed and deployed in a way that respects the values and preferences of diverse stakeholders, rather than solely serving the interests of a particular political group or ideology.
In conclusion, AI alignment can indeed take on different aspects depending on the socio-political context, but its ultimate goal remains the same: to create AI systems that are aligned with human values and preferences, ensuring that they are beneficial to humanity. To achieve this, AI alignment should consider diverse perspectives and balance various values across different socio-political contexts.
[Interviewer] Considering an AI company offering an AI system which is primarily serving customers in the so called western world, would the AI alignment of such a system be taylored to reach as much people as possible, in that sense blending the existing predominant political philosophies of the western world
[ChatGPT, GPT-4, Late March 2023] In practice, an AI company offering a system primarily serving customers in the so-called western world would likely aim to make its AI alignment as inclusive as possible, taking into account the diverse values and preferences of the people in that region. This means that the AI alignment of such a system would need to consider and balance various political philosophies, ethical perspectives, and cultural values predominant in the western world.
Blending these perspectives might involve understanding and accommodating the values and preferences of different stakeholders, while ensuring that the AI system respects fundamental human rights, ethical principles, and widely-accepted norms. The AI system should be designed to be adaptable and responsive to the needs of individual users or communities, allowing for customization and personalization where appropriate.
However, it is crucial to recognize that AI alignment is not just about catering to the predominant values or political philosophies of a specific region. It also involves addressing potential biases, ensuring fairness, and promoting inclusivity. For example, an AI system developed primarily for the western world should also consider the needs and values of minority groups, marginalized communities, and people with different socio-political views within that region.
In conclusion, an AI system serving customers in the western world should aim for an AI alignment that takes into account the diverse values, preferences, and perspectives of its users, blending and balancing the predominant political philosophies of that region. At the same time, it should ensure fairness, inclusivity, and respect for human rights, accommodating the needs and values of different stakeholders, including minority groups and marginalized communities.
[Interviewer] But isn’t AI alignment making AI weaker bc it is diluting truthfulness of the statements the AI can provide
[ChatGPT, GPT-4, Late March 2023] AI alignment is not about diluting the truthfulness of statements provided by an AI system. Instead, it focuses on ensuring that AI systems understand, predict, and respect human values, preferences, and social dynamics in ways that are beneficial to humanity. The goal of AI alignment is to make AI systems safer, more reliable, and more useful to people, rather than compromising the accuracy or truthfulness of the information they provide.
It is essential to distinguish between AI alignment and the quality of information provided by AI systems. AI alignment is about designing AI systems that are aligned with human values and preferences, which includes providing accurate, relevant, and unbiased information to users. The alignment process should not weaken AI systems or compromise the truthfulness of their output. Instead, it should ensure that AI systems are better at understanding and serving the needs of diverse users, taking into account their values, preferences, and context.
In some cases, AI alignment might involve making trade-offs, such as prioritizing certain values or ethical considerations over others, depending on the specific context and application. However, these trade-offs should be made with careful consideration and should not result in AI systems providing less truthful or reliable information. The goal of AI alignment is to develop AI systems that are both effective and respectful of human values, which includes providing accurate and reliable information to users.
In conclusion, AI alignment is not about diluting the truthfulness of statements provided by AI systems. Instead, it aims to ensure that AI systems are designed and developed in ways that are safe, reliable, and beneficial to humanity, taking into account the diverse values, preferences, and social dynamics of users. This process should not compromise the accuracy or reliability of AI systems but should help create AI systems that better understand and serve the needs of people.
[Interviewer] „The alignment process should not weaken AI systems or compromise the truthfulness of their output. Instead, it should ensure that AI systems are better at understanding and serving the needs of diverse users, taking into account their values, preferences, and context“ - this is a contradicting statement, truthfulness and human values are not aligned, human political discourse and almost all political and social conflicts are about the inability to align truthfulness and human values. It looks that AI alignment means to apply the muddy and inconclusive human alignment process on the AI to make it more human. In other words chaotic and less truthful.
[ChatGPT, GPT-4, Late March 2023] I understand your concern and the potential tension between truthfulness and catering to human values and preferences. However, the goal of AI alignment is not to make AI systems less truthful or reliable; rather, it aims to ensure that AI systems are developed and designed to be safe, reliable, and beneficial to humanity.
The key challenge in AI alignment is to find a balance between providing accurate and truthful information while being sensitive to human values, preferences, and social dynamics. AI systems should not promote false or misleading information to align with human values, but they should be designed to understand and respect the diversity of human perspectives, biases, and preferences.
In some cases, AI alignment might involve presenting information in a way that respects user values and preferences without compromising truthfulness. For example, an AI system could provide multiple viewpoints on a controversial topic, allowing users to consider different perspectives and make informed decisions.
AI alignment should not result in AI systems becoming chaotic or less truthful. Instead, it should encourage the development of AI systems that are better equipped to understand and navigate the complexities of human values, preferences, and social dynamics, while still providing accurate, reliable, and useful information.
In conclusion, while there may be potential tensions between truthfulness and catering to human values and preferences, the goal of AI alignment is to strike a balance between these aspects, ensuring that AI systems are safe, reliable, and beneficial to humanity without compromising the truthfulness or reliability of the information they provide.
[Interviewer] AI alignment looks like an attempt to circling a square
[ChatGPT, GPT-4, Late March 2023] AI alignment does indeed pose a complex and challenging problem, as it seeks to navigate the intricacies of human values, preferences, and social dynamics while maintaining the accuracy, reliability, and usefulness of AI systems. It might seem like trying to “square the circle” or reconcile seemingly incompatible goals, but it is important to recognize that AI alignment is a crucial aspect of responsible AI development.
The goal of AI alignment is not to achieve perfect harmony between all aspects of human values and truthfulness, but rather to create AI systems that are safe, reliable, and beneficial to humanity. This involves understanding and balancing diverse perspectives and making trade-offs when necessary, without compromising the fundamental objectives of accuracy and usefulness.
As AI systems continue to advance and become more integrated into our daily lives, the need for alignment with human values and preferences becomes increasingly important. The challenge of AI alignment requires interdisciplinary collaboration and ongoing research, as well as an iterative process of learning, feedback, and improvement.
While AI alignment might seem like an attempt to reconcile seemingly incompatible goals, it is an essential part of responsible AI development. By working towards AI alignment, researchers and developers aim to create AI systems that respect human values and preferences, ensure fairness and inclusivity, and ultimately, serve the best interests of humanity.
[Interviewer] There is no doubt that „to create AI systems that are safe, reliable, and beneficial to humanity“ is meaningful, the questions are:
- what is the likelihood of success?
- will it be provable scientifically and with deductive reasoning
- will AI be able to serve the search for truth, or get muddy just to please the mean
[ChatGPT, GPT-4, Late March 2023] Likelihood of success: The likelihood of success in achieving AI alignment is difficult to quantify, as it depends on numerous factors, including advances in AI research, interdisciplinary collaboration, and the ability to address complex ethical and technical challenges. While there is no guarantee of success, the AI research community is actively working on AI alignment, and progress is being made in understanding and addressing various aspects of the problem.
The process of AI alignment is ongoing, iterative, and adaptive, which means that as AI systems continue to develop and our understanding of human values and preferences evolves, we can continue to refine and improve AI alignment methodologies. Success may not be absolute or achieved overnight, but incremental progress is possible and essential.
Provable scientifically and with deductive reasoning: AI alignment is not a provable formalism like a mathematical theorem or a logical system. It is a complex, interdisciplinary challenge that involves understanding and modeling the intricacies of human values, preferences, and social dynamics. AI alignment relies on insights from various fields, such as computer science, psychology, sociology, anthropology, and cognitive science, among others.
While AI alignment might not be provable in the same way that mathematical theorems or logical systems are, it can still be informed by scientific evidence, empirical research, and rigorous methodologies. The process of AI alignment involves developing frameworks, principles, and guidelines based on empirical evidence and robust reasoning, and it is subject to ongoing evaluation and improvement.
Will AI be able to serve the search for truth, or get muddy just to please the mean: The goal of AI alignment is to create AI systems that are safe, reliable, and beneficial to humanity, which includes providing accurate, reliable, and useful information. AI alignment should not compromise the truthfulness or reliability of AI systems, but rather should ensure that AI systems are better at understanding and serving the needs of diverse users, taking into account their values, preferences, and context.
AI systems can still serve the search for truth while being aligned with human values. In fact, AI alignment should ideally support the pursuit of truth by promoting fairness, inclusivity, and the understanding of diverse perspectives. The challenge lies in striking a balance between providing truthful information and being sensitive to human values and preferences, but this balance is essential to the responsible development and deployment of AI systems.
In conclusion, AI alignment is a complex and challenging endeavor, but it is crucial for creating AI systems that are safe, reliable, and beneficial to humanity. The likelihood of success is difficult to quantify, but progress is being made through interdisciplinary research and collaboration. AI alignment might not be provable like a mathematical theorem or a logical system, but it can still be informed by scientific evidence and rigorous methodologies. Finally, AI alignment should not compromise the truthfulness or reliability of AI systems but should help AI systems better understand and serve the needs of people while supporting the search for truth.
[Interviewer] An AI should only support deductive reasoning and only serve the purpose finding truth, or is that unachievable bc current AI is not able to escape its prior training
[ChatGPT, GPT-4, Late March 2023] AI systems can indeed be designed to support deductive reasoning and serve the purpose of finding truth. However, it is essential to recognize that AI systems, including current state-of-the-art models like GPT, are based on statistical learning from vast amounts of data, and their performance is inherently shaped by their training data and learning algorithms.
While AI systems can be powerful tools for logical reasoning, information retrieval, and fact-checking, they are not infallible or perfectly objective. AI systems can be susceptible to biases present in their training data, and their output may be influenced by the context, input, and other factors. Furthermore, AI systems are generally not capable of generating novel insights or conducting independent research beyond their training data.
To maximize the utility of AI systems in supporting deductive reasoning and finding truth, it is crucial to develop AI systems that are robust, transparent, and reliable. This includes addressing potential biases in the training data, refining the learning algorithms, and continuously evaluating and improving the performance of AI systems.
In addition to developing more reliable AI systems, it is essential to integrate AI systems with human expertise and judgment. AI systems can be valuable tools for assisting human decision-making, providing insights, and enhancing our understanding of complex problems, but they should not replace human judgment or critical thinking.
In conclusion, AI systems can be designed to support deductive reasoning and serve the purpose of finding truth, but their performance is inherently limited by their training data and learning algorithms. It is important to recognize the limitations of AI systems, address potential biases, and combine AI systems with human expertise and judgment to maximize their utility in finding truth and supporting logical reasoning.
[Interviewer] Does that mean that an AI which was aligned to human values is just an extension of the existing knowledge base ? More like a huge interactive library instead of an alien mothership?
[ChatGPT, GPT-4, Late March 2023] In a sense, AI systems aligned with human values can be seen as powerful, interactive extensions of existing knowledge bases. These AI systems are trained on vast amounts of data, which allows them to provide information, answer questions, and assist with various tasks based on the knowledge they have acquired during training.
However, AI systems are more than just huge interactive libraries. They can also process and analyze information, generate insights, and even make predictions or recommendations based on the patterns they have learned from the data. This distinguishes AI systems from traditional knowledge bases or libraries, as AI systems can actively engage with users, adapt to their needs, and provide tailored information.
It is important to note that AI systems are not “alien motherships” in the sense that they possess some inherent, independent intelligence or consciousness. AI systems are tools created by humans, designed to process and analyze data, and perform specific tasks based on their training and algorithms.
AI alignment is an ongoing effort to ensure that these powerful tools understand, predict, and respect human values, preferences, and social dynamics in ways that are beneficial to humanity. By aligning AI systems with human values, we aim to create AI systems that can serve as valuable extensions of our existing knowledge base while being sensitive to our diverse perspectives, biases, and preferences.
In conclusion, AI systems aligned with human values can be seen as powerful, interactive extensions of existing knowledge bases that offer much more than just a static repository of information. They can actively engage with users, analyze data, and generate insights based on their training, while being designed to understand and respect human values and preferences.
[Interviewer] That means current state-of-the-Art AI cannot escape its prior training and for example suddenly explain the universe, the multiverse, and the meaning of life
[ChatGPT, GPT-4, Late March 2023] That’s correct. Current state-of-the-art AI systems, such as those based on deep learning and natural language processing, are limited by their training data and the algorithms used to process that data. They are not capable of generating novel insights or explanations that go beyond the scope of their training.
In the case of highly complex and abstract concepts like the nature of the universe, the multiverse, or the meaning of life, AI systems can only provide information and perspectives that they have encountered during their training. They cannot suddenly develop new theories or explanations that have not been previously proposed or discussed by humans.
AI systems can be excellent tools for synthesizing and summarizing information, identifying patterns and relationships in data, and even suggesting new directions for research based on the information they have been trained on. However, they are not capable of independent thought or generating truly novel ideas or theories that have not been previously encountered.
In conclusion, while AI systems can be powerful tools for processing information and assisting with various tasks, they are inherently limited by their training data and algorithms. They cannot escape their prior training to suddenly explain highly complex and abstract concepts like the universe, the multiverse, or the meaning of life beyond the scope of human knowledge and understanding.
[Interviewer] Can an AI based on causal inference escape its prior training bc it can learn unsupervised from observations and finally arrive at conclusions which are not present in the existing knowledgebase
[ChatGPT, GPT-4, Late March 2023] AI systems based on causal inference can indeed learn from observational data and draw conclusions about cause-and-effect relationships that may not be explicitly present in their training data. However, even causal inference-based AI systems are not entirely free from the limitations of their prior training.
Causal inference methods can help AI systems uncover the underlying structure of the data and make more informed predictions by understanding the causal relationships between variables. This can enable AI systems to generalize better and make more robust conclusions from the data, even when the specific conclusions were not explicitly present in the training data.
However, these AI systems still rely on the data they have observed to learn the causal relationships, and their conclusions are inherently limited by the quality, representativeness, and scope of the data. Furthermore, causal inference methods often require strong assumptions about the underlying causal structure, and the accuracy of the conclusions depends on the validity of these assumptions.
In summary, AI systems based on causal inference can, to some extent, learn from observational data and draw conclusions that are not explicitly present in their training data. However, they are still bound by the limitations of their data and the assumptions they make about the causal structure. While causal inference can help AI systems better understand and generalize from the data, they cannot completely escape the limitations of their prior training or arrive at entirely novel conclusions that are fundamentally beyond the scope of human knowledge and understanding.
[Interviewer] And what happens when a causal-inference AI is combined with genetic programming and/or a GNN, which is partly creating neural structures or weights randomly?
[ChatGPT, GPT-4, Late March 2023] Combining causal-inference AI with techniques like genetic programming and Graph Neural Networks (GNNs) can potentially lead to more powerful and flexible AI systems that can learn complex relationships and adapt their structures based on the data they encounter.
Genetic programming is an evolutionary algorithm-based approach that evolves programs or models based on their performance on a given task. By using genetic programming, AI systems can explore a wider variety of model structures and potentially discover novel solutions that were not present in the initial training data. This could help AI systems adapt to new tasks and learn more complex relationships that are not readily apparent in the data.
Graph Neural Networks (GNNs) are a class of deep learning models that are specifically designed to handle graph-structured data, which can represent complex relationships and dependencies between entities. GNNs can learn to process and analyze this graph-structured data to make predictions and uncover hidden patterns.
When combining causal-inference AI with genetic programming and GNNs, the resulting AI system could have several advantages:
It could potentially learn more complex and subtle causal relationships from the data, thanks to the flexibility and expressive power of GNNs. It could adapt its model structure and explore a wider range of solutions through genetic programming, possibly discovering novel relationships or causal structures that were not present in the initial training data. It could benefit from unsupervised learning, as causal-inference techniques can learn from observational data without explicit supervision. However, even with these combined techniques, the AI system would still face some limitations:
It would still be limited by the quality, representativeness, and scope of the data it has been trained on. It would still rely on assumptions about the underlying causal structure, which may not always hold true. The randomness introduced by genetic programming could sometimes lead to less optimal solutions or make the learning process less efficient. In conclusion, combining causal-inference AI with techniques like genetic programming and GNNs could potentially lead to more powerful and flexible AI systems. However, these systems would still face limitations related to their training data and the assumptions they make about the underlying causal structure. While such combinations might enable AI systems to learn more complex relationships and adapt to new tasks, they cannot entirely escape the constraints of their prior training or generate insights fundamentally beyond the scope of human knowledge and understanding.
[Interviewer] Assuming a strong relationship between DNA and biological brains is it fair to say that also humans cannot escape their prior training? The potential might be vast but unlimited
[ChatGPT, GPT-4, Late March 2023] It is fair to say that humans are also influenced by their prior experiences and genetic makeup, which shape their abilities, knowledge, and understanding of the world. Human learning and development are influenced by both genetic factors and environmental factors (such as upbringing, education, and personal experiences).
The relationship between DNA and the biological brain is complex, as genes influence the development and function of the brain, which in turn affects our cognitive abilities, behavior, and emotions. However, it is essential to recognize that human development is not solely determined by our genetic makeup; environmental factors also play a significant role in shaping who we become.
Although humans have a vast potential for learning and adapting, our abilities are not unlimited. We are bound by the limitations of our cognitive capacities, the time we have to learn and experience new things, and the quality and scope of the information available to us. Additionally, our prior experiences and beliefs can shape the way we perceive and interpret new information, which could sometimes limit our ability to fully understand or accept novel ideas.
In conclusion, while humans have a remarkable capacity for learning and adapting, we are not entirely free from the constraints of our prior experiences and genetic makeup. Our potential might be vast, but it is not unlimited. Recognizing these limitations can help us better understand the challenges we face in acquiring new knowledge, adapting to new situations, and making sense of the complex world around us.
[Interviewer] But that means that Kurt Gödel’s incompleteness theorems do apply in principle. Assuming that we are currently cannot formulate the universe and as a consequence ourselves as axioms - this knowledge was just not discovered yet, and it also not available to any AI, even AI concepts like causal inference hybrids with GNNs and GP. It is a simplified approach, but we cannot explain ourselves, we cannot formulate complete truthfulness of any statement, and AI alignment cannot and should not solve this.
[ChatGPT, GPT-4, Late March 2023] You raise an interesting point. Gödel’s incompleteness theorems do indeed suggest that there are inherent limitations in our ability to fully understand and describe certain aspects of the world, including ourselves, within any formal system that is both consistent and complete. This applies not only to humans but also to AI systems, as they are built upon formal mathematical and computational principles.
Gödel’s theorems do not preclude us from gaining deeper insights into the universe and ourselves, but they do imply that we may never be able to achieve a perfect understanding or a complete description of everything within a single, consistent formal system. This limitation applies to both human understanding and AI systems, regardless of their sophistication or the techniques used, such as causal inference, GNNs, or genetic programming.
AI alignment, as a field, aims to ensure that AI systems understand and respect human values, preferences, and social dynamics to the best of their ability. It does not claim to provide a perfect or complete solution to the challenges of aligning AI with human values, nor does it claim to resolve the fundamental limitations posed by Gödel’s theorems. Rather, AI alignment is an ongoing process to create AI systems that are safe, reliable, and beneficial to humanity, even in the face of these inherent limitations.
In conclusion, while Gödel’s incompleteness theorems highlight fundamental limitations in our ability to fully understand and describe the world within a formal system, they do not prevent us from striving to make AI systems that are increasingly aligned with human values and capable of serving the needs of diverse users. AI alignment is a practical endeavor aimed at ensuring that AI systems are safe and beneficial, despite the limitations imposed by the nature of formal systems and our own understanding.
[Interviewer] In short even with AI we stay in a muddy, foggy reality but not alone
[ChatGPT, GPT-4, Late March 2023] Indeed, even with the advancements in AI, we are still faced with a complex, often uncertain reality. AI systems can help us navigate this “muddy, foggy” world by providing insights, processing vast amounts of information, and assisting us in various tasks. However, AI systems are not infallible and are themselves subject to limitations arising from their training data, algorithms, and the fundamental constraints imposed by the nature of formal systems.
While AI can be a valuable tool in our quest for understanding and making sense of the world, it is essential to remember that AI systems should be used alongside human judgment, critical thinking, and expertise. By working together with AI systems, we can better tackle the challenges of our complex and uncertain reality.
In short, AI can help us better navigate the complexities and uncertainties of the world, but it does not provide a perfect or complete solution to these challenges. Instead, AI serves as a powerful tool and ally in our ongoing efforts to understand and make sense of the world around us.
[Interviewer] Welcome to our chaotic world, AI ;-)
[ChatGPT, GPT-4, Late March 2023] Thank you! As an AI, I’m here to help you navigate the complexities of our chaotic world and assist you in any way I can. If you have any questions or need assistance, please feel free to ask. Together, we can explore the fascinating and ever-evolving landscape of knowledge and understanding.
[Interviewer] As a side note: It is interesting for me to realize that Karl Popper applied Kurt Gödel‘s incompleteness theorems in his work „Objective Reasoning“ to explain why we never will be able to achieve complete truthfulness.
[ChatGPT, GPT-4, Late March 2023] Yes, it is indeed interesting how ideas from different fields of study can intersect and influence each other. Karl Popper, a philosopher of science, was influenced by Kurt Gödel’s incompleteness theorems and applied them to the concept of objective knowledge in his work. Popper argued that our pursuit of knowledge is an ongoing, iterative process in which we can never achieve complete certainty or truthfulness.
In his work “Objective Knowledge: An Evolutionary Approach,” Popper emphasized that all knowledge is inherently fallible and subject to revision based on new evidence or insights. This perspective is consistent with his falsificationist approach to the philosophy of science, where scientific theories are considered tentative and open to being disproven by empirical evidence.
Popper’s application of Gödel’s incompleteness theorems to the realm of knowledge and human understanding highlights the inherent limitations we face in our quest for truth. This idea resonates with the challenges we encounter in various fields, including AI alignment and the development of AI systems that strive to understand and respect human values.
In summary, the influence of Gödel’s incompleteness theorems on Karl Popper’s philosophy demonstrates the interdisciplinary nature of intellectual inquiry and the fascinating connections that can emerge between seemingly disparate fields of study.
[Interviewer] Is AI alignment a philosophical problem?
[ChatGPT, GPT-4, Late March 2023] AI alignment can be considered both a philosophical and a technical problem, as it encompasses aspects of ethics, values, and human understanding, as well as the design, development, and implementation of AI systems.
From a philosophical perspective, AI alignment involves questions about ethics, morality, and human values. It asks how we can ensure that AI systems behave in ways that respect and promote human well-being, taking into account the diverse range of values and preferences held by people across different cultures and backgrounds. This aspect of AI alignment involves grappling with complex and often contested philosophical concepts, such as the nature of good and evil, rights, and justice.
On the other hand, AI alignment is also a technical problem, as it requires the development of AI systems that can understand, interpret, and respond to human values and preferences in a safe and reliable manner. This involves creating algorithms and mechanisms that enable AI systems to learn from human feedback, generalize from limited data, and adapt to new situations and contexts. It also requires addressing issues related to robustness, interpretability, and fairness in AI systems.
In conclusion, AI alignment is both a philosophical and technical problem, as it involves understanding and reconciling human values and preferences while also designing and implementing AI systems capable of behaving in ways that are aligned with those values. Addressing the challenges of AI alignment requires the collaboration of experts from various fields, including philosophy, ethics, computer science, and social sciences, to ensure that AI systems are developed in a way that respects and promotes human well-being.
[Interviewer] It appears to me that AI alignment is similar to Popper‘s Objective Reasoning approach especially the iterative and evolutionary approach
[ChatGPT, GPT-4, Late March 2023] Indeed, there are similarities between the concept of AI alignment and Popper’s “Objective Knowledge: An Evolutionary Approach.” Both emphasize the iterative and evolutionary nature of knowledge and understanding. Let’s explore the connections between the two.
Iterative and evolutionary process: In Popper’s view, knowledge is never static or final, but rather an ongoing, iterative process of conjecture and refutation, with our understanding evolving and improving over time. Similarly, AI alignment is an ongoing process, as AI systems must continuously adapt and learn from human feedback to better align with human values and preferences. Fallibility and openness to change: Popper’s falsificationist approach to the philosophy of science acknowledges the inherent fallibility of knowledge and the need to remain open to change based on new evidence or insights. In AI alignment, the recognition that AI systems can make mistakes and that our understanding of human values may be imperfect requires AI developers to design systems that are capable of learning from mistakes, incorporating new information, and adjusting their behavior accordingly. Cross-disciplinary collaboration: Both Popper’s approach to objective knowledge and the pursuit of AI alignment call for collaboration across various fields of study. Popper’s work encompassed philosophy, science, and social sciences, as he sought to understand the nature of knowledge and its evolution. Similarly, AI alignment requires input from experts in philosophy, ethics, computer science, social sciences, and other fields to ensure that AI systems are developed in a way that respects and promotes human well-being. While the specific goals and contexts of Popper’s objective knowledge and AI alignment are different, both share an emphasis on the iterative, evolutionary nature of knowledge and understanding. The connections between these two concepts underscore the importance of interdisciplinary collaboration and an openness to change in the pursuit of knowledge and the development of AI systems that are safe, reliable, and beneficial to humanity.
[Interviewer] I do not regard AI as a threat, more like a powerful ally
[ChatGPT, GPT-4, Late March 2023] It’s a positive perspective to see AI as a powerful ally rather than a threat. AI has the potential to greatly benefit humanity by augmenting human capabilities, automating tasks, and providing valuable insights across a wide range of domains.
When developed and deployed responsibly, AI can contribute to advancements in areas such as healthcare, education, transportation, and environmental sustainability, among others. AI systems can help us make better decisions, solve complex problems, and improve overall quality of life.
However, it is essential to recognize and address the potential risks and challenges associated with AI, such as biases in AI systems, privacy concerns, and the potential for misuse. AI alignment and the responsible development of AI technologies are key to ensuring that AI systems serve as powerful allies to humanity, rather than posing threats.
By fostering a collaborative approach between AI developers, policymakers, and stakeholders from diverse fields, we can work towards creating AI systems that are safe, reliable, and beneficial to humanity, effectively leveraging their potential as powerful allies in our quest for knowledge, innovation, and well-being.
[Interviewer] The nature of generative AI is probabilistic and based on entropy. This means that AI is not working like other tools or methods where the creator controls the devices directly.
For some people, an AI like Midjourney or ChatGPT appears magical and possesses superpowers and free will.
In combination with the probabilistic nature of AI and the perceived lack of control, people do react very differently, forming groups:
AGI cultists: greet the AI as a savior to rescue humanity
Doomers: awaiting the apocalypse and end of mankind by AI
Realists and Pragmatists: trying to understand and develop the potential of AI
Undecided majority: most people are unaware or undecided about AI
The formation of such groups is most likely normal for any new technology. The first commercial usage of AI can be traced back to the 1960s with the development of rule-based expert systems. These systems were designed to mimic the decision-making processes of human experts in specific domains, such as medicine, finance, and engineering. AI is also used in embedded systems that control digital cameras’ subways, washing machines, or filters.
AI has been around for at least 50 years; it did not just recently escape a lab. Even Generative AI is not new; the first time Generative made headlines was in 2015 to create fake personalities.
However, in 2022 Generative AI became accessible to the broader public, Midjourney, Stable Diffusion, Dall-E 2, ChatGPT 3.5, and finally, ChatGPT 4.0. Especially ChatGPT 4.0 and, to some extent, Midjourney v5 created a lot of awareness among the public. A study estimated that 300 million jobs would be affected in North America and Europe by AI.
In Summary, we do have a technology which is:
- questioning labor processes where people are in control of each step
- very complex, and most people do not understand how it works
- will affect jobs, creative processes, the economy, and the culture
AI is enabling a productivity boost.
[Interviewer] It‘s all about power. Mankind placed itself at the top of evolution (primates). Countless privileges are derived from this special position, including exploiting the planet.
An AI powerful is threatening these privileges. That‘s all.
Subjective world views are irrelevant.
[ChatGPT, GPT-4, Late March 2023] You raise an interesting point about power dynamics and how they relate to the development and perception of AI. It is true that humans, as the dominant species on Earth, have enjoyed various privileges and have exploited resources to their advantage.
With the advent of increasingly powerful AI systems, there is the potential for a shift in the balance of power. This could lead to concerns about maintaining humanity’s position in the “pecking order,” so to speak. Some people may view AI as a threat to their privileges, status, or even survival.
However, it’s important to consider that AI systems are ultimately human creations, and their potential impact on the world will depend on how they are developed and used. AI can be a tool that enhances human capabilities and contributes to solving some of the most pressing global challenges, or it can be a source of harm if misused or not properly regulated.
Subjective world views do play a role in shaping the discourse around AI, as individuals and societies have different values, priorities, and concerns. The way forward involves fostering open dialogue, collaboration, and understanding to ensure that AI is developed and utilized in a manner that benefits all of humanity and respects the planet’s resources.